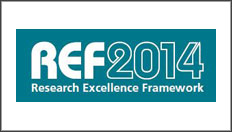
Steven Hill of HEFCE has posted �an overview of the work HEFCE are currently commissioning which they are hoping will build a robust evidence base for research assessment� in LSE Impact Blog 12(17) 2014 entitled
Time for REFlection: HEFCE look ahead to provide rounded evaluation of the REF
Let me add a suggestion, updated for REF2014, that I have made before (unheeded):
Scientometric predictors of research performance need to be validated by showing that they have a high correlation with the external criterion they are trying to predict. The UK Research Excellence Framework (REF) -- together with the growing movement toward making the full-texts of research articles freely available on the web -- offer a unique opportunity to test and validate a wealth of old and new scientometric predictors, through multiple regression analysis: Publications, journal impact factors, citations, co-citations, citation chronometrics (age, growth, latency to peak, decay rate), hub/authority scores, h-index, prior funding, student counts, co-authorship scores, endogamy/exogamy, textual proximity, download/co-downloads and their chronometrics, tweets, tags, etc.) can all be tested and validated jointly, discipline by discipline, against their REF panel rankings in REF2014. The weights of each predictor can be calibrated to maximize the joint correlation with the rankings. Open Access Scientometrics will provide powerful new means of navigating, evaluating, predicting and analyzing the growing Open Access database, as well as powerful incentives for making it grow faster.
Harnad, S. (2009)
Open Access Scientometrics and the UK Research Assessment Exercise.
Scientometrics 79 (1)
(Also in Proceedings of 11th Annual Meeting of the International Society for Scientometrics and Informetrics 11(1), pp. 27-33, Madrid, Spain. Torres-Salinas, D. and Moed, H. F., Eds. 2007)
See also:
The Only Substitute for Metrics is Better Metrics (2014)
and
On Metrics and Metaphysics (2008)
REF2014 gives the 2014 institutional and departmental rankings based on the 4 outputs submitted.
That is then the criterion against which the many other metrics I list below can be jointly validated, through multiple regression, to initialize their weights for REF2020, as well as for other assessments. In fact, open access metrics can be � and will be � continuously assessed, as open access grows. And as the initialized weights of the metric equation (per discipline) are optimized for predictive power, the metric equation can replace the peer rankings (except for periodic cross-checks and updates) -- or at least supplement it.
Single metrics can be abused, but not only can abuses be named and shamed when detected, but it becomes harder to abuse metrics when they are part of a multiple, inter-correlated vector, with disciplinary profiles of their normal interactions: someone dispatching a robot to download his papers would quickly be caught out when the usual correlation between downloads and later citations fails to appear. Add more variables and it gets even harder.
In a weighted vector of multiple metrics like the sample I had listed, it�s no use to a researcher if told in advance that for REF2020 the metric equation will be the following, with the following weights for their particular discipline:
REF2020Rank =
w1(pubcount) + w2(JIF) + w3(cites) +w4(art-age) + w5(art-growth) + w6(hits) + w7(cite-peak-latency) + w8(hit-peak-latency) + w9(citedecay) + w10(hitdecay) + w11(hub-score) + w12(authority+score) + w13(h-index) + w14(prior-funding) +w15(bookcites) + w16(student-counts) + w17(co-cites + w18(co-hits) + w19(co-authors) + w20(endogamy) + w21(exogamy) + w22(co-text) + w23(tweets) + w24(tags), + w25(comments) + w26(acad-likes) etc. etc.
The potential list could be much longer, and the weights can be positive or negative, and varying by discipline.
"The man who is ready to prove that metric knowledge is wholly impossible� is a brother metrician with rival metrics��