Update Jan 1, 2010: See Gargouri, Y; C Hajjem, V Larivière, Y Gingras, L Carr,T Brody & S Harnad (2010) “Open Access, Whether Self-Selected or Mandated, Increases Citation Impact, Especially for Higher Quality Research”
Update Feb 8, 2010: See also "Open Access: Self-Selected, Mandated & Random; Answers & Questions"
Summary: Antelman et al. (2005) hand-tested the accuracy of the algorithm that Hajjem et al.'s (2005) software robot used to to trawl the web and automatically identify Open Access (OA) and Non-Open-Access (NOA) articles (references derived from the ISI database). Antelman et al. found much lower accuracy than Hajjem et al. Had reported. Hajjem et al. have now re-done the hand-testing on a larger sample (1000) in Biology, and demonstrated that Hajjem et al.'s original estimate of the robot's accuracy was much closer to the correct one. The discrepancy was because both Antelman et al. And Hajjem et al had hand-checked a sample other than the one the robot was sampling. Our present sample, identical with what the robot saw, yielded: d' 2.62, bias 0.68, true OA 93%, false OA 12%. We also checked whether the OA citation advantage (the ratio of the average citation counts for OA articles to the average citation counts for NOA articles in the same journal/issue) was an artifact of false OA: The robot-based OA citation Advantage of OA over NOA for this sample [(OA-NOA)/NOA x 100] was 70%. We partitioned this into the ratio of the citation counts for true (93%) OA articles to the NOA articles versus the ratio of the citation counts for the false (12%) "OA" articles. The "false OA" advantage for this 12% of the articles was 33%, so there is definitely a false OA Advantage bias component in our results. However, the true OA advantage, for 93% of the articles, was 77%. So in fact, we are underestimating the true OA advantage.
Previous AmSci Topic Thread:
"Manual Evaluation of Algorithm Performance on Identifying OA" (Dec 2005)
References cited:
Antelman, K., Bakkalbasi, N., Goodman, D., Hajjem, C. and Harnad, S. (2005) Evaluation of Algorithm Performance on Identifying OA. Technical Report, North Carolina State University Libraries, North Carolina State University.
Hajjem, C., Harnad, S. and Gingras, Y. (2005) Ten-Year Cross-Disciplinary Comparison of the Growth of Open Access and How it Increases Research Citation Impact. IEEE Data Engineering Bulletin 28(4) pp. 39-47.
In an unpublished study, Antelman et al. (2005) hand-tested the accuracy of the algorithm that Hajjem et al.'s (2005) software robot used to identify Open Access (OA) and Non-Open-Access (NOA) articles in the ISI database. Antelman et al. found much lower accuracy (d' 0.98, bias 0.78, true OA 77%, false OA 41%), with their larger sample of nearly 600 (half OA, half NOA) in Biology (and even lower, near-chance performance in Sociology, sample size 600, d' 0.11, bias 0.99, true OA 53% false OA 49%) compared to Hajjem et al., who had with their smaller Biology sample of 200, found: d' 2.45, beta 0.52, true OA 93%, false OA 16%.
Hajjem et al. have now re-done the hand-testing on a still larger sample (1000) in Biology, and we think we have identified the reason for the discrepancy, and demonstrated that Hajjem et al.'s original estimate of the robot's accuracy was closer to the correct one.
The discrepancy was because Antelman et al. were hand-checking a sample other than the one the robot was sampling: The templates are the ISI articles. The ISI bibliographic data (author, title, etc.) for each article is first used to automatically trawl the web with search engines looking for hits, and then the robot applies its algorithm to the first 60 hits, calling the article "OA" if the algorithm thinks it has found at least one OA full-text among the 60 hits sampled, and NOA if it does not find one.
Antelman et al. did not hand-check these same 60 hits for accuracy, because the hits themselves were not saved; the only thing recorded was the robot's verdict on whether a given article was OA or NOA. So Antelman et al. generated another sample -- with different search engines, on a different occasion -- for about 300 articles that the robot had previously identified as having an OA version in its sample, and 300 for which it had not found an OA version in its sample; Antelman et al.'s hand-testing found much lower accuracy.
Hajjem et al.'s first test of the robot's accuracy made the very same mistake of hand-checking a new sample instead of saving the hits, and perhaps it yielded higher accuracy only because the time difference between the two samples was much smaller (but the search engines were again not the same ones used). Both accuracy hand-tests were based on incommensurable samples.
Testing the robot's accuracy in this way is analogous to testing the accuracy of an instant blood test for the presence of a disease in a vast number of villages by testing a sample of 60 villagers in each (and declaring the disease to be present in the village (OA) if a positive case is detected in the sample of 60, NOA otherwise) and then testing the accuracy of the instant test against a reliable incubated test, but doing this by picking
another sample of 60 from 100 of the villages that had previously been identified as "OA" based on the instant test and 100 that had been identified as "NOA." Clearly, to test the accuracy of the first, instant test, the second test ought to have been performed on the very same
individuals on which the first test had been performed, not on another sample based only on the overall outcome of the first test, at the whole-village level.
So when we hand-checked the actual hits (URLs) that the robot had identified as "OA" or "NOA" in our Biology sample of 1000, saving all the hits this time, the robot's accuracy was again much higher: d' 2.62, bias 0.68, true OA 93%, false OA 12%.
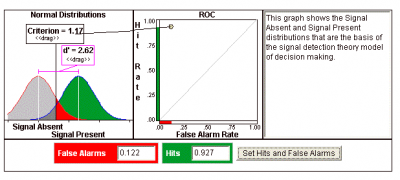
All this merely concerned the robot's accuracy in detecting true OA. But our larger hand-checked sample now also allowed us to check whether the OA citation advantage (the ratio of the average citation counts for OA articles to the average citation counts for NOA articles in the same journal/issue) was an artifact of false OA:
We accordingly had the robot's estimate of the OA citation Advantage of OA over NOA for this sample [(OA-NOA)/NOA x 100 = 70%], and we could now partition this into the ratio of the citation counts for true (93%) OA articles to the NOA articles (false NOA was very low, and would have worked against an OA citation advantage) versus the ratio of the citation counts for the false (12%) "OA" articles. The "false OA" advantage for this 12% of the articles was 33%, so there is definitely a false OA Advantage bias component in our results. However, the true OA advantage, for 93% of the articles, was 77%. So in fact, we are underestimating the OA advantage.
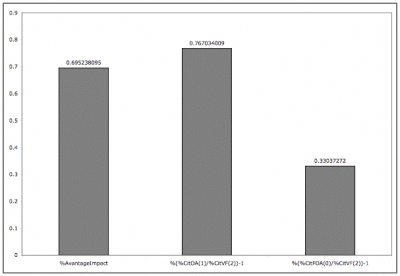
As explained in previous postings on the American Scientist topic thread, the purpose of the robot studies is not to get the most accurate possible estimate of the current percentage of OA in each field we study, nor even to get the most accurate possible estimate of the size of the OA citation Advantage. The advantage of a robot over much more accurate hand-testing is that we can look at a much larger sample, and faster -- indeed, we can test all of the articles in all the journals in each field in the ISI database, across years. Our interest at this point is in nothing more accurate than a rank-ordering of %OA as well as %OA citation Advantage across fields and years. We will nevertheless tighten the algorithm a little; the trick is not to make the algorithm so exacting for OA as to make it start producing substantially more false NOA errors, thereby weakening its overall accuracy for %OA as well as %OA advantage.
Stevan Harnad & Chawki Hajjem